Robots can gain from their current circumstance without human intercession through a blend of cutting edge innovations, including AI, computerized reasoning (man-made intelligence), and sensor frameworks. These advancements empower robots to see, investigate, and adjust to their environmental factors independently. This cycle, frequently called independent learning, is pivotal for robots working in unique or unusual conditions.

To gain from their current circumstance, robots depend on sensors to assemble information. These sensors can distinguish different ecological information sources, like light, sound, temperature, strain, and movement. For example, cameras give visual information, mouthpieces catch sound, and material sensors imitate a feeling of touch. These tangible data sources permit robots to see their environmental factors progressively, framing the establishment for independent learning.
Machine Learning Algorithms
AI, especially support learning, is critical to empowering robots to learn independently. In support learning, a robot collaborates with its current circumstance, making moves and getting criticism as remunerations or punishments. Over the long run, the robot utilizes this criticism to refine its way of behaving and further develop execution. For instance, a robot exploring a labyrinth may at first make irregular developments, however it steadily learns the ideal way by recognizing activities that lead to progress.
Directed learning and unaided learning can likewise assume a part. In regulated learning, robots dissect marked datasets to perceive designs, for example, object acknowledgment or discourse handling. In solo learning, robots find examples or connections inside information without predefined names, which is especially valuable in investigating obscure conditions.
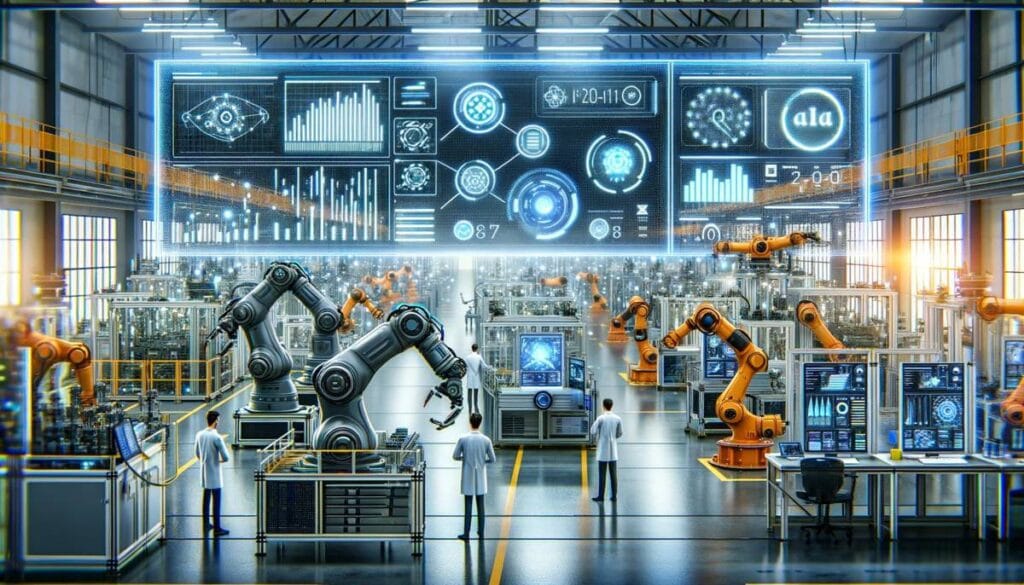
Adaptive Systems and Neural Networks
Robots outfitted with fake brain organizations can reproduce the manner in which the human cerebrum processes data. These organizations permit robots to distinguish complex examples and go with choices in view of related involvements. By consistently refreshing their models, robots can adjust to new conditions without requiring unequivocal programming. For example, a modern robot could recognize mileage in hardware by gaining from unpretentious changes in sound or vibration designs.
Swarm Knowledge and Cooperative Learning
Robots can likewise advance by and large through swarm knowledge, a strategy propelled by friendly bugs like honey bees and insects. In this methodology, different robots share data about their current circumstance, upgrading their aggregate information. For instance, drones planning a fiasco zone can share information, empowering them to make an exhaustive guide more productively than a solitary robot.
The Role of Autonomy
By joining sensors, AI, brain organizations, and cooperation, robots accomplish a degree of independence that permits them to adjust, improve, and pursue choices without human mediation. This capacity is fundamental in applications like independent vehicles, automated investigation, and high level assembling.
All in all, robots gain from their current circumstance through a mix of tangible discernment, information driven calculations, and versatile frameworks. These advances engage robots to work autonomously, preparing for progressions in mechanical technology and computer based intelligence across assorted fields.